AWS Certified Machine Learning - Specialty (MLS-C01) Exam Guide
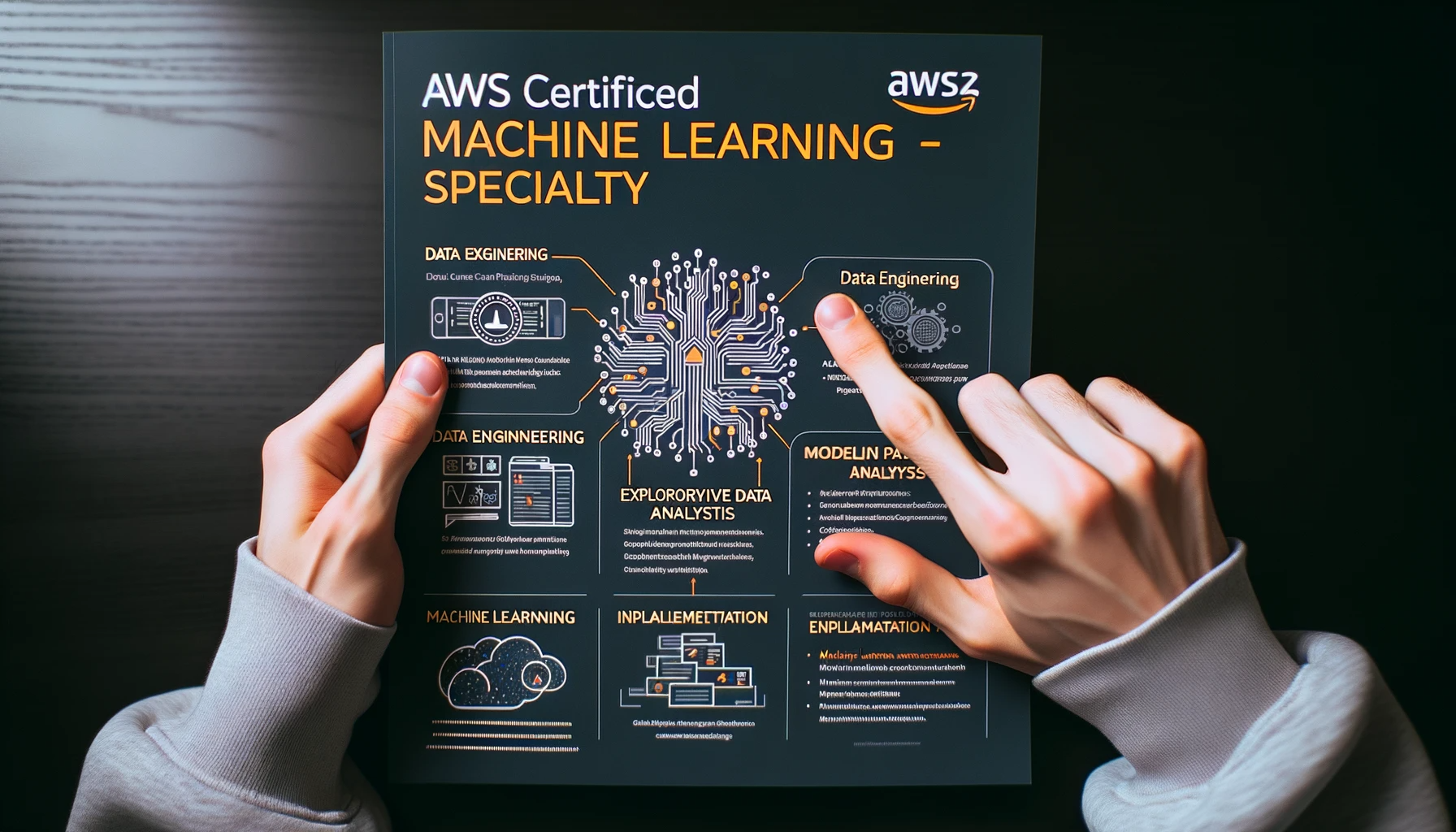
This guide provides a comprehensive overview of the AWS Certified Machine Learning - Specialty (MLS-C01) exam. It includes details on the exam's structure, content domains, task statements, and the relevant AWS services and features. It also includes an appendix with additional resources.
Introduction #
The AWS Certified Machine Learning - Specialty (MLS-C01) exam is intended for individuals who perform an artificial intelligence/machine learning (AI/ML) development or data science role. The exam validates a candidate’s ability to design, build, deploy, optimize, train, tune, and maintain ML solutions for given business problems by using the AWS Cloud.
The exam also validates a candidate’s ability to complete the following tasks:
- Select and justify the appropriate ML approach for a given business problem.
- Identify appropriate AWS services to implement ML solutions.
- Design and implement scalable, cost-optimized, reliable, and secure ML solutions.
Target candidate description #
The target candidate should have 2 or more years of experience developing, architecting, and running ML or deep learning workloads in the AWS Cloud.
Recommended AWS knowledge #
The target candidate should have the following AWS knowledge:
- The ability to express the intuition behind basic ML algorithms
- Experience performing basic hyperparameter optimization
- Experience with ML and deep learning frameworks
- The ability to follow model-training best practices
- The ability to follow deployment best practices
- The ability to follow operational best practices
Knowledge that is out of scope for the target candidate #
The following list contains knowledge that the target candidate is not expected to have. This list is non-exhaustive. Knowledge in the following areas is out of scope for the exam:
- Extensive or complex algorithm development
- Extensive hyperparameter optimization
- Complex mathematical proofs and computations
- Advanced networking and network design
- Advanced database, security, and DevOps concepts
- DevOps-related tasks for Amazon EMR
Refer to the Appendix for a list of technologies and concepts that might appear on the exam, a list of in-scope AWS services and features, and a list of out-of-scope AWS services and features.
Exam content #
Response types #
There are two types of questions on the exam:
- Multiple choice: Has one correct response and three incorrect responses (distractors)
- Multiple response: Has two or more correct responses out of five or more response options
Select one or more responses that best complete the statement or answer the question. Distractors, or incorrect answers, are response options that a candidate with incomplete knowledge or skill might choose. Distractors are generally plausible responses that match the content area. Unanswered questions are scored as incorrect; there is no penalty for guessing. The exam includes 50 questions that affect your score.
Unscored content #
The exam includes 15 unscored questions that do not affect your score. AWS collects information about performance on these unscored questions to evaluate these questions for future use as scored questions. These unscored questions are not identified on the exam.
Exam results #
The AWS Certified Machine Learning - Specialty (MLS-C01) exam has a pass or fail designation. The exam is scored against a minimum standard established by AWS professionals who follow certification industry best practices and guidelines.
Your results for the exam are reported as a scaled score of 100–1,000. The minimum passing score is 750. Your score shows how you performed on the exam as a whole and whether you passed. Scaled scoring models help equate scores across multiple exam forms that might have slightly different difficulty levels.
Your score report could contain a table of classifications of your performance at each section level. The exam uses a compensatory scoring model, which means that you do not need to achieve a passing score in each section. You need to pass only the overall exam.
Each section of the exam has a specific weighting, so some sections have more questions than other sections have. The table of classifications contains general information that highlights your strengths and weaknesses. Use caution when you interpret section-level feedback.
Content outline #
This exam guide includes weightings, content domains, and task statements for the exam. This guide does not provide a comprehensive list of the content on the exam. However, additional context for each task statement is available to help you prepare for the exam.
The exam has the following content domains and weightings:
- Domain 1: Data Engineering (20% of scored content)
- Domain 2: Exploratory Data Analysis (24% of scored content)
- Domain 3: Modeling (36% of scored content)
- Domain 4: Machine Learning Implementation and Operations (20% of scored content)
Domain 1: Data Engineering #
Task Statement 1.1: Create data repositories for ML.
- Identify data sources
- content and location
- primary sources such as user data.
- Determine storage mediums
- databases
- Amazon S3
- Amazon Elastic File System [Amazon EFS]
- Amazon Elastic Block Store [Amazon EBS]
Task Statement 1.2: Identify and implement a data ingestion solution.
- Identify data job styles and job types (for example, batch load, streaming).
- Orchestrate data ingestion pipelines (batch-based ML workloads and streaming-based ML workloads).
- Amazon Kinesis
- Amazon Kinesis Data Firehose
- Amazon EMR
- AWS Glue
- Amazon Managed Service for Apache Flink
- Schedule jobs.
Task Statement 1.3: Identify and implement a data transformation solution.
- Transform data in transit:
- Extract, transform, and load (ETL)
- AWS Glue
- Amazon EMR
- AWS Batch
- Handle ML-specific data by using MapReduce
- Apache Hadoop
- Apache Spark
- Apache Hive
Domain 2: Exploratory Data Analysis #
Task Statement 2.1: Sanitize and prepare data for modeling.
- Identify and handle missing data, corrupt data, and stop words.
- Format, normalize, augment, and scale data.
- Determine whether there is sufficient labeled data.
- Identify mitigation strategies.
- Use data labelling tools
- Amazon Mechanical Turk
Task Statement 2.2: Perform feature engineering.
- Identify and extract features from datasets
- text
- speech
- image
- public datasets.
- Analyze and evaluate feature engineering concepts
- binning
- tokenization
- outliers
- synthetic features
- one-hot encoding
- reducing dimensionality of data
Task Statement 2.3: Analyze and visualize data for ML.
- Create graphs
- scatter plots
- time series
- histograms
- box plots
- Interpret descriptive statistics
- correlation
- summary statistics
- p-value
- Perform cluster analysis
- hierarchical
- diagnosis
- elbow plot
- cluster size
Domain 3: Modeling #
Task Statement 3.1: Frame business problems as ML problems.
- Determine when to use and when not to use ML.
- Know the difference between supervised and unsupervised learning.
- Select from:
- classification
- regression
- forecasting
- clustering
- recommendation models.
Task Statement 3.2: Select the appropriate model(s) for a given ML problem.
- XGBoost, logistic regression, k-means, linear regression, decision trees, random forests, RNN, CNN, ensemble, transfer learning - Express the intuition behind models.
Task Statement 3.3: Train ML models.
- Split data between training and validation (for example, cross validation).
- Understand optimization techniques for ML training (for example, gradient decent, loss functions, convergence).
- Choose appropriate compute resources (for example GPU or CPU, distributed or non-distributed).
- Choose appropriate compute platforms (Spark or non-Spark).
- Update and retrain models.
- Batch or real-time/online
Task Statement 3.4: Perform hyperparameter optimization.
- Perform regularization.
- Drop out
- L1/L2
- Perform cross validation.
- Initialize models.
- Understand neural network architecture (layers and nodes), learning rate, and activation functions.
- Understand tree-based models (number of trees, number of levels).
- Understand linear models (learning rate).
Task Statement 3.5: Evaluate ML models.
- Avoid overfitting or underfitting.
- Detect and handle bias and variance.
- Evaluate metrics (area under curve [AUC]-receiver operating characteristics [ROC], accuracy, precision, recall, Root Mean Square Error [RMSE], F1 score).
- Interpret confusion matrices.
- Perform offline and online model evaluation (A/B testing).
- Compare models by using metrics (for example, time to train a model, quality of model, engineering costs).
- Perform cross validation.
Domain 4: Machine Learning Implementation and Operations #
Task Statement 4.1: Build ML solutions for performance, availability, scalability, resiliency, and fault tolerance.
- Log and monitor AWS environments.- AWS CloudTrail and Amazon CloudWatch- Build error monitoring solutions.
- Deploy to multiple AWS Regions and multiple Availability Zones.
- Create AMIs and golden images.
- Create Docker containers.
- Deploy Auto Scaling groups.
- Rightsize resources (for example, instances, Provisioned IOPS, volumes).
- Perform load balancing.
- Follow AWS best practices.
Task Statement 4.2: Recommend and implement the appropriate ML services and features for a given problem.
- ML on AWS (application services)
- Amazon Polly
- Amazon Lex
- Amazon Transcribe
- Understand AWS service quotas.
- Determine when to build custom models and when to use Amazon SageMaker built-in algorithms.
- Understand AWS infrastructure (for example, instance types) and cost considerations.
- Use Spot Instances to train deep learning models by using AWS Batch.
Task Statement 4.3: Apply basic AWS security practices to ML solutions.
- AWS Identity and Access Management (IAM)
- S3 bucket policies
- Security groups
- VPCs
- Encryption and anonymization
Task Statement 4.4: Deploy and operationalize ML solutions.
- Expose endpoints and interact with them.
- Understand ML models.
- Perform A/B testing.
- Retrain pipelines.
- Debug and troubleshoot ML models.
- Detect and mitigate drops in performance.
- Monitor performance of the model.
Appendix #
Technologies and concepts that might appear on the exam #
The following list contains technologies and concepts that might appear on the exam. This list is non-exhaustive and is subject to change. The order and placement of the items in this list is no indication of their relative weight or importance on the exam:
- Ingestion/collection
- Processing/ETL
- Data analysis/visualization
- Model training
- Model deployment/inference
- Operationalizing ML
- AWS ML application services
- Language relevant to ML (for example, Python, Java, Scala, R, SQL)
- Notebooks and integrated development environments (IDEs)
In-scope AWS services and features #
The following list contains AWS services and features that are in scope for the exam. This list is non-exhaustive and is subject to change. AWS offerings appear in categories that align with the offerings’ primary functions:
Analytics:
- Amazon Athena
- Amazon EMR
- AWS Glue
- Amazon Kinesis
- Amazon Kinesis Data Firehose
- Amazon Kinesis Data Streams
- Amazon Managed Service for Apache Flink
- Amazon QuickSight
Compute:
- AWS Batch
- Amazon EC2
- AWS Lambda
Containers:
- Amazon Elastic Container Registry (Amazon ECR)
- Amazon Elastic Container Service (Amazon ECS)
- Amazon Elastic Kubernetes Service (Amazon EKS)
- AWS Fargate
Database:
- Amazon Redshift
Internet of Things:
- AWS IoT Greengrass
Machine Learning:
- Amazon Comprehend
- AWS Deep Learning AMIs (DLAMI)
- AWS DeepLens
- Amazon Forecast
- Amazon Fraud Detector
- Amazon Lex
- Amazon Mechanical Turk
- Amazon Polly
- Amazon Rekognition
- Amazon SageMaker
- Amazon Textract
- Amazon Transcribe
- Amazon Translate
Management and Governance:
- AWS CloudTrail
- Amazon CloudWatch
Networking and Content Delivery:
- Amazon VPC
Security, Identity, and Compliance:
- AWS Identity and Access Management (IAM)
Storage:
- Amazon Elastic Block Store (Amazon EBS)
- Amazon Elastic File System (Amazon EFS)
- Amazon FSx
- Amazon S3
Out-of-scope AWS services and features #
The following list contains AWS services and features that are out of scope for the exam. This list is non-exhaustive and is subject to change. AWS offerings that are entirely unrelated to the target job roles for the exam are excluded from this list: Out-of-scope AWS services and features include the following:
Analytics:
- AWS Data Pipeline
Machine Learning:
- AWS DeepRacer
- Amazon Machine Learning (Amazon ML)